AI Safety Data Solutions
Many areas of business impact safety performance - all are underpinned by data. Our solutions improve, harness and leverage your safety data through AI and advanced technologies to deliver deeper insights, support your safety data journey, and contribute to safer workplaces.
THE INTEGRATED SAFETY DATA MODEL
All Libero AI safety data solutions employ our Integrated Safety Data Model (ISDM) to transform the safety data landscape and support your journey from data capture to actionable insights, ultimately helping you deliver safer workplaces.
This approach provides a new visual context and enables you to quickly identify emerging areas of safety and risk concerns.
ISDM Process:
-
Define: Identify and understand key risks / hazards and regulations.
-
Plan: Establishing conditions and controls to mitigate identified risks.
-
Work: Perform work in a dynamic environment while continuously adjusting methods for emerging risks.
-
Monitor: Track performance, spot unsafe trends, and validate controls.
-
Action: Consolidate issues, assign corrective measures, and follow through.
-
Outcomes: Analyse failures, near misses, and general performance to create a continuous improvement and feedback loop.
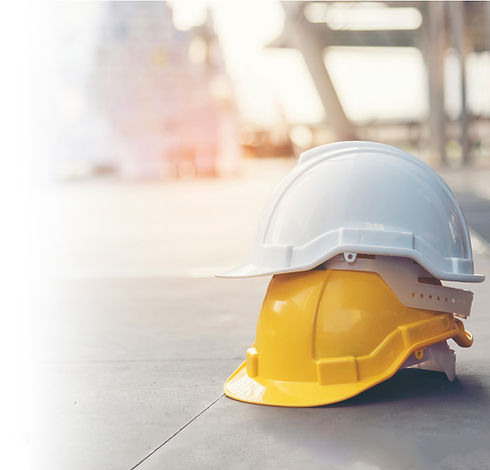
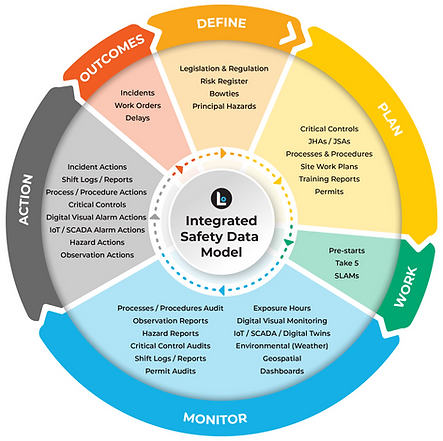
THE AI SAFETY DATA JOURNEY

PHASE 1
IMPROVING SAFETY DATA
Improving data quality is the essential first step to improved safety and risk performance.
We help you improve data quality on capture to reduce inconsistencies in incident, hazard and observation reporting, leading to faster, more precise root-cause analyses and safer workplaces.
The phase utilises the following AI / analysis methods:
-
AI Assistant
-
Natural Language Processing
-
Large Language Models
-
Visualisation
REAL-WORLD APPLICATION EXAMPLES:
-
Safety forms use live checklists, NLP, and LLM prompts to parse free-text fields and categorise inputs, reducing input time and upholding criteria checks to produce high quality submissions.
-
Update historical incident, hazard and observation data where it is missing categorisations to provide quality data to harness and leverage.
PHASE 2
HARNESSING
YOUR SAFETY DATA
Once your safety data quality at capture is improved, we help harness it.
Unifying data allows for comprehensive trend spotting, revealing the often-hidden links between hazard reports, maintenance records, and operational tasks.
The phase can utilise the following:
-
Natural Language Processing / Large Language Models
-
Machine Learning
-
Agentic AI
-
Knowledge Graphs
-
Topic Modelling
REAL-WORLD APPLICATION EXAMPLES:
-
Map relationships between hazards, behaviors, and equipment failures to surface non-obvious connections that might span multiple departments or sites.
-
Automate the tagging of new incident reports, update relevant risk registers, and proactively route high-priority issues to the correct safety personnel.
-
Group similar hazard descriptions or failure modes together, allowing safety teams to see overarching themes in a large volume of text.

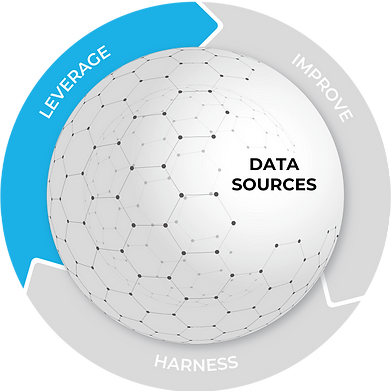
PHASE 3
LEVERAGING
YOUR SAFETY DATA
Once your safety data is harnessed, our AI solutions help leverage it to provide actionable insights.
When AI models continuously scan incident data and sensor readings they can detect subtle patterns such as slight increases in rates of equipment collision alerts and operator feedback about damage to mine walls and mesh - therefore indicating emerging risks.
The phase can utilise the following:
-
Machine Learning
-
Natural Language Processing
-
Visualisation
-
Bayesian Inference
-
Agentic AI
-
Root-cause Discovery
REAL-WORLD APPLICATION EXAMPLES:
-
Calculate the probability that a particular hazard will occur based on past occurrences, current sensor trends, and real-time shifts in operating conditions.
-
Automate early warning tasks by issuing alerts to relevant managers or creating work orders the moment a threshold is breached (e.g., temperature spikes in a critical pump).
-
Examine consistent clusters of anomalies i.e. repeated motor overheating or sporadic sensor failures, to pinpoint underlying problems that drive hazard formation.